Generative AI vs Predictive AI: Understanding the Differences
Generative AI and Predictive AI Are Emerging Trends Generative and predictive artificial intelligence are two forms of artificial intelligence which have gained increasing prominence within the technology industry. Predictive AI analyzes past data to predict future events or trends; it’s instrumental in industries or roles which rely heavily on data predictions. By contrast, generative AI focuses more on content creation – such as text documents, videos, images, code or music creation.
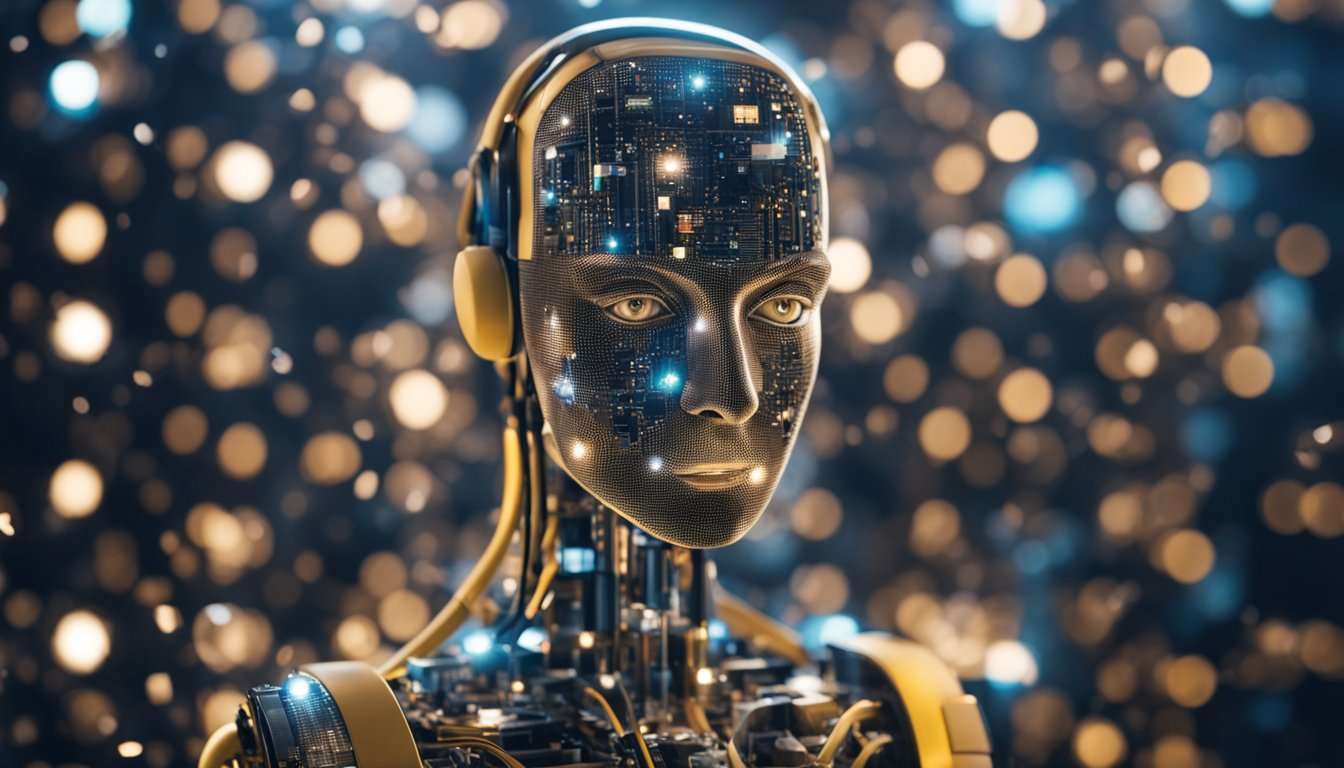
As artificial intelligence (AI) technologies advance, interest among enterprises for various generative AI forms has gradually grown. Now, with increased capabilities available through artificial intelligence technologies, however, more businesses are turning to them to automate content production and save marketers time. At the same time, predictive AI provides actionable insights for marketing strategies and optimization.
As AI capabilities increase, we must comprehend the differences between generative AI and predictive AI. In this article, we explore their use cases, limitations, and ethical considerations before discussing what lies ahead for both types of AIs.
Key Takeaways
- Generative AI specializes in content production while predictive AI makes accurate predictions from historical data.
- Predictive AI is particularly useful for industries or roles that rely on data-driven predictions, while generative AI provides automated content creation services to save marketers time.
- As AI technology evolves, it’s vital that individuals understand the differences and applications between generative and predictive AI.
Related Posts:
Understanding Generative AI
Generative AI (Generative Artificial Intelligence) is an artificial intelligence technique designed to generate new ideas. As part of machine learning, this branch specializes in creativity and innovation. Generative AI can be applied for various tasks such as content production, design, or creation using neural networks and machine learning algorithms that specialize in producing fresh material.
Generative AI’s most significant advantage lies in its capacity to produce unique content – something beneficial for industries such as marketing and advertising, where companies need new ways to reach their target audience. Generative AI can create anything from marketing collateral and music compositions all the way down to code writing!
Generative AI relies on deep learning techniques such as GANs and VAEs for its outputs, which allow it to produce synthetic data that resembles real-world information that can then be used by machine learning models to generate new content.
Recent advances in generative AI include large language models (LLMs). These models can reproduce human-like text generation, profoundly impacting content production and software development.
Overall, generative AI is a highly potent tool for creativity and innovation. It can transform industries such as marketing, advertising and software development by helping companies produce unique and new content at scale.
Related Posts:
Understanding Predictive AI
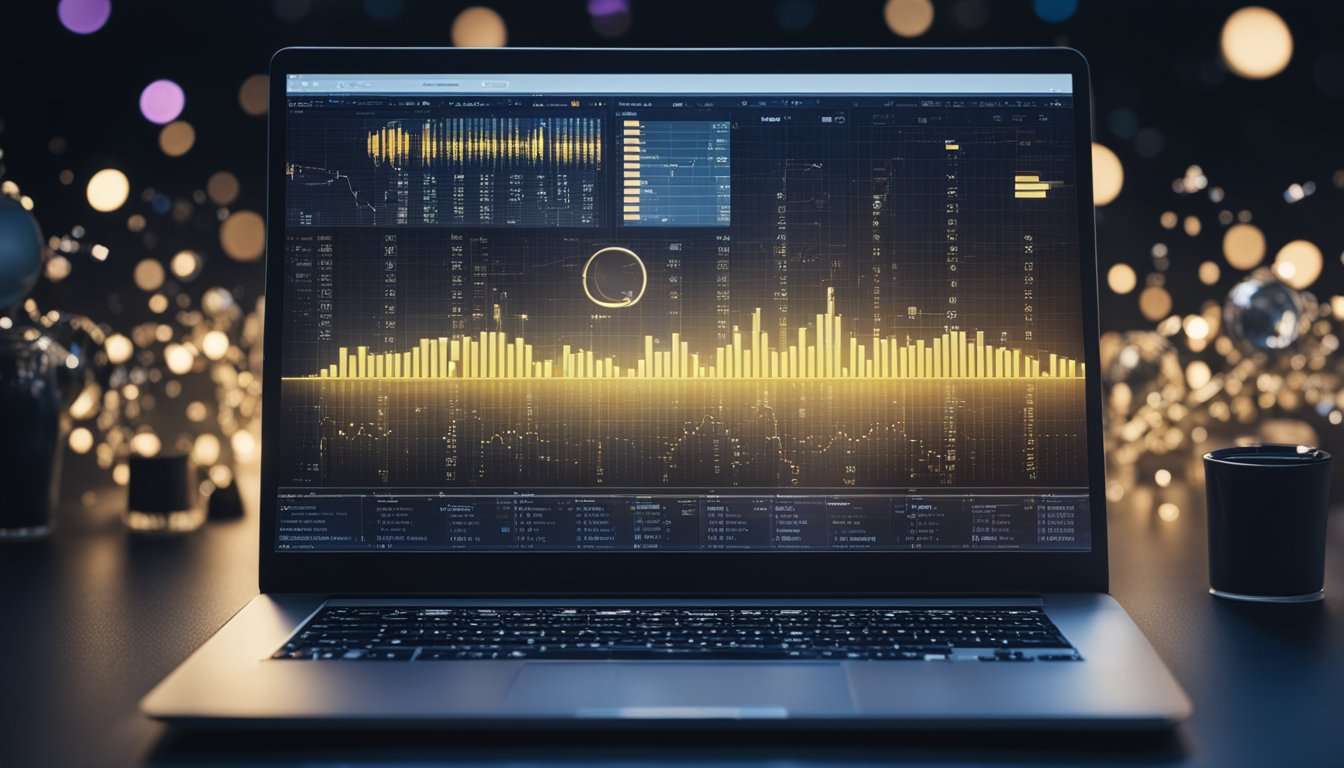
Predictive AI (AI) is a subfield of artificial intelligence (AI) using historical data to predict or forecast events that have yet to happen. Predictive AI uses machine learning techniques that involve training algorithms to recognize patterns within data sets to use these relationships to make predictions of upcoming events.
Predictive AI has found widespread application across finance, healthcare and marketing industries. It helps optimize business processes, enhance decision-making abilities and automate tasks more efficiently than traditional methods can.
Predictive AI relies heavily on statistical algorithms and machine learning models to analyze data and make predictions. These models can be trained using linear regression, decision trees, or neural networks.
Accuracy is of utmost importance in predictive AI models. Their performance depends on the quality and relevance of data used to train it; bias could also pose a problem, as models could be trained on biased sets that lead to inaccurate predictions.
To maximize the accuracy of predictive AI models, algorithms used for training must be carefully selected while ensuring training data remains current and pertinent. Regular monitoring and optimization must also occur to maintain optimal model performance over time.
Predictive AI is a powerful tool for making accurate predictions and forecasts based on historical data. Through machine learning algorithms and statistical models, businesses can gain insightful customer behaviour patterns, optimize business processes and make data-driven decisions that will benefit from predictive AI solutions.
Generative and Predictive AI in Different Industries
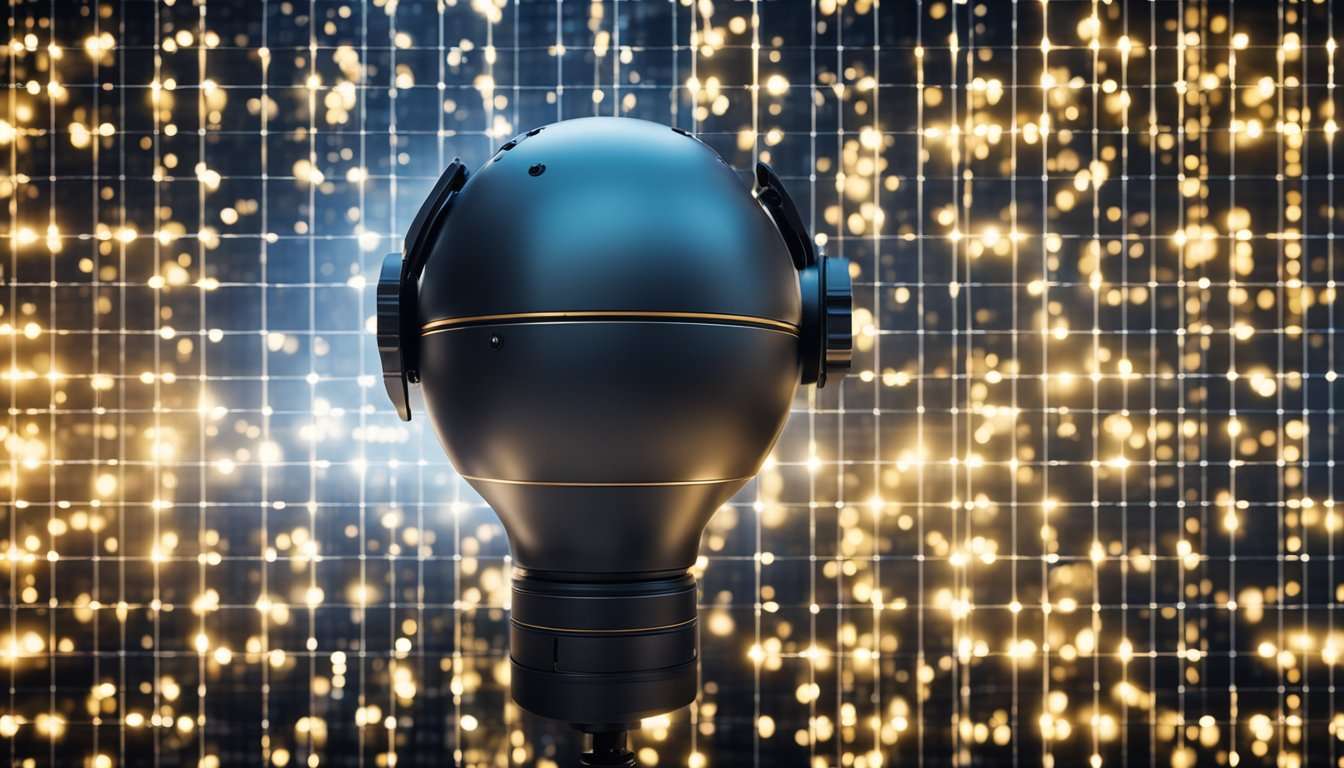
Artificial Intelligence (AI) has made significant strides across various industries, such as healthcare, finance, supply chain management, retail banking, logistics and manufacturing. Generative AI and predictive AI both play critical roles within these fields of activity.
Predictive AI is used in healthcare to diagnose diseases and predict future health outcomes, for instance, by analyzing medical images to detect cancerous tumours. Generative AI, on the other hand, creates synthetic data sets which can improve accuracy by providing more diverse input data sets for training predictive AI models.
Predictive AI has many uses in finance, such as fraud detection, credit scoring and risk management. Predictive AI models can analyze financial transactions to detect fraudulent activity. At the same time, Generative AI creates synthetic financial data sets for training predictive AI models to improve accuracy by providing more diverse input data sets.
Supply chain predictive AI applications include demand forecasting, inventory optimization and route optimization. Predictive AI models can analyze past sales data to predict future demand. At the same time, Generative AI creates synthetic supply chain data sets to train predictive AI models more accurately by providing more diverse inputs.
Retail retailers leverage predictive AI for targeted marketing, inventory control and price optimization. Predictive AI models can analyze customer data to suggest products tailored to them. Generative AI can also generate synthetic retail datasets to train predictive AI models more accurately by providing more diverse data sets to train on.
Banking applications of predictive AI include fraud detection, credit scoring, and customer service. Predictive AI models can analyze financial transactions to detect fraudulent activity. At the same time, Generative AI creates synthetic banking data sets, which improve predictive AI model accuracy by providing more diverse data sets to train them on.
Logistics applications of predictive AI include route optimization, delivery tracking and warehouse management. Predictive AI models can analyze traffic data to optimize delivery routes. At the same time, Generative AI creates synthetic logistic data sets to train predictive AI models – potentially improving their accuracy by providing more diverse input data sets.
Predictive AI in manufacturing can be used for quality control, predictive maintenance and supply chain optimization. Predictive AI models can analyze sensor data to predict equipment failure. Meanwhile, Generative AI creates synthetic manufacturing data sets, which help train predictive AI models more accurately by providing more diverse data sets for training purposes.
Overall, both predictive AI and generative AI have various applications across industries. Predictive AI analyzes historical data to make future forecasts. At the same time, generative AI creates synthetic data sets to bolster predictive models’ accuracy.
Use Cases of Generative and Predictive AI
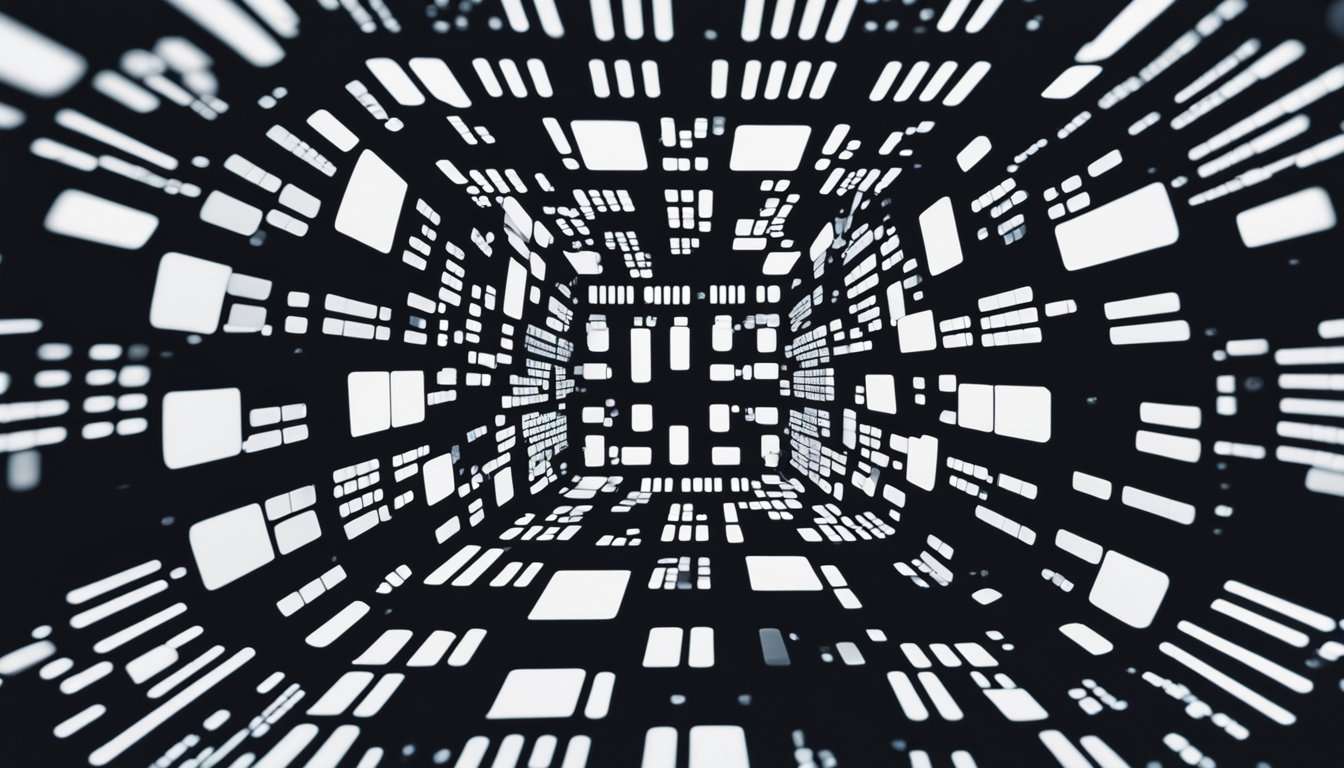
Generative AI and predictive AI both have distinct applications and usage scenarios.
Generative AI Use Cases
Generative AI is designed to generate new ideas and produce original content. It is suitable for use across industries that rely heavily on content creation – including marketing, sales, and innovation. Generative AI may create campaigns or personalized customer experiences, make product or service recommendations, or create marketing plans.
Generative AI can also be applied in customer service as a virtual chatbot for fast services, simulating human-like conversations and quickly providing solutions to customer inquiries.
Generative AI also plays an essential role in problem-solving and simulations. Simulations created using this technology can test various scenarios and predict outcomes; such a solution would prove invaluable for drug research or inventory management purposes.
Predictive AI Use Cases
Predictive AI analyses historical data to predict future events or trends, making predictions for their occurrence or development. Predictive AI has numerous applications across industries that need demand forecasting, such as banking and finance, or churn prediction to detect customers who might churn away from services or products so companies can take measures to keep customers from leaving prematurely.
Predictive AI can also help businesses increase efficiency in business operations. It can be utilized for inventory management to predict demand and optimize stock levels and supply chain operations to predict delivery times and optimize routes.
Predictive AI gives business leaders the data-driven decisions needed to make intelligent business decisions. By analyzing large volumes of data, predictive AI provides valuable insights that allow business leaders to make well-informed decisions.
Both generative AI and predictive AI offer distinct uses and applications; the former is great for creative tasks and developing innovative solutions, while predictive AI excels at analyzing historical data and forecasting future events or trends.
Limitations and Ethical Considerations of Generative and Predictive AI
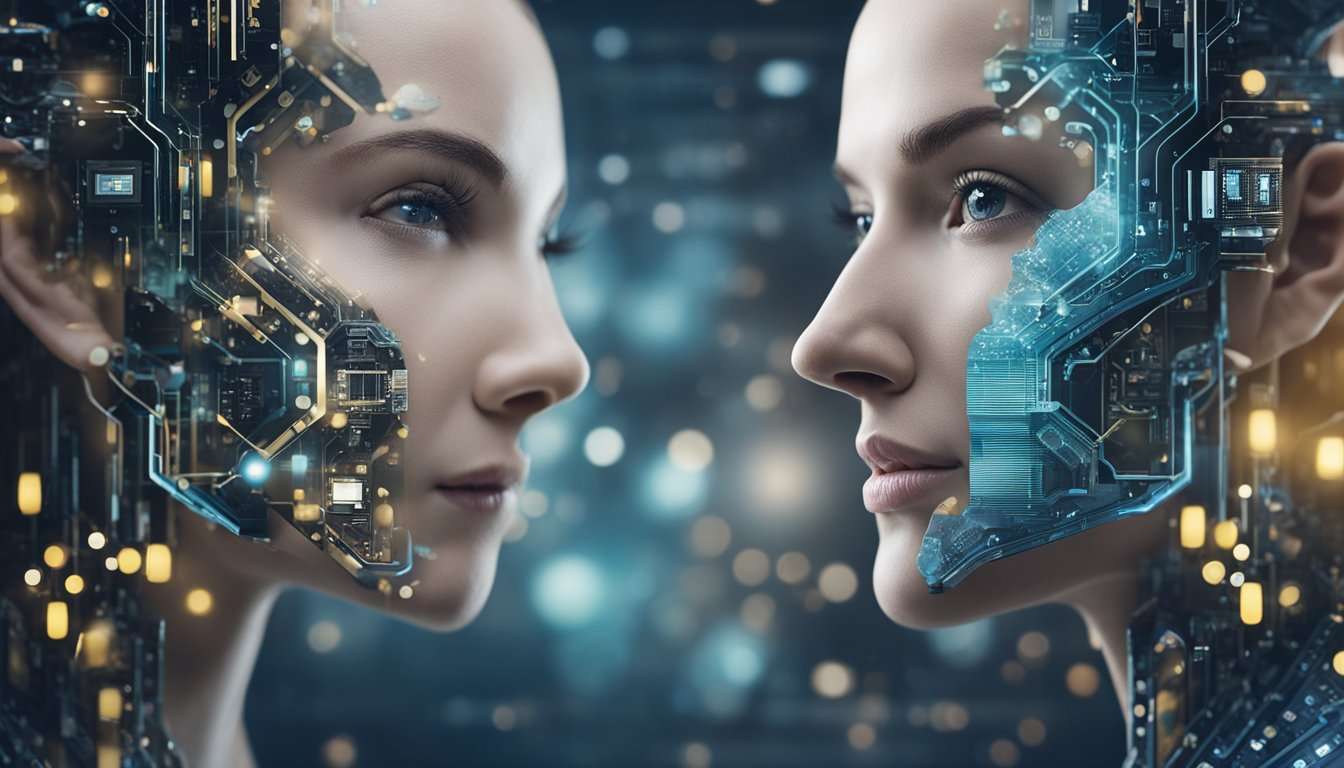
As with any technology, AI technologies come with challenges and ethical considerations that must be considered when used for commercial applications.
Limitations
One of the critical drawbacks of generative AI is that it requires an extensive amount of data for training the models. Without sufficient inputs, models may not produce accurate or fair results, and generative AI may not suit specific applications, such as real-time decision-making applications.
On the other hand, predictive AI may have limitations regarding its ability to make accurate predictions. Predictive models rely on training data; incomplete or biased input could make predictions inaccurate, and predictive AI may need help to predict events not included in its initial training set.
Ethics
Ethics Both generative and predictive AI raise ethical concerns. For generative AI specifically, one primary problem lies with potential bias in data used for training models – should such discrimination exist within training data, any results produced could potentially have adverse social and economic ramifications.
Predictive AI raises ethical concerns, particularly around its potential to discriminate. If predictive models are used in hiring decisions, for instance, they could unfairly favour certain groups and reinforce patterns of discrimination by reinforcing inequalities between people.
Misinformation
Misinformation, Both predictive and generative AI, creates the potential for misinformation; inaccurate models could produce information that harms individuals and society. Furthermore, generative AI could generate fake news or spread disinformation.
Future Trends
As Artificial Intelligence technology progresses, generative and predictive AI may become more advanced and accurate; however, keeping a close eye on their ethical implications and any potential harm associated with these technologies remains essential.
Overall, AI technologies pose opportunities and ethical concerns that must be carefully managed as their use grows. Therefore, taking an active and thoughtful approach towards their usage will help ensure they are used responsibly and ethically.
The Future of Generative and Predictive AI
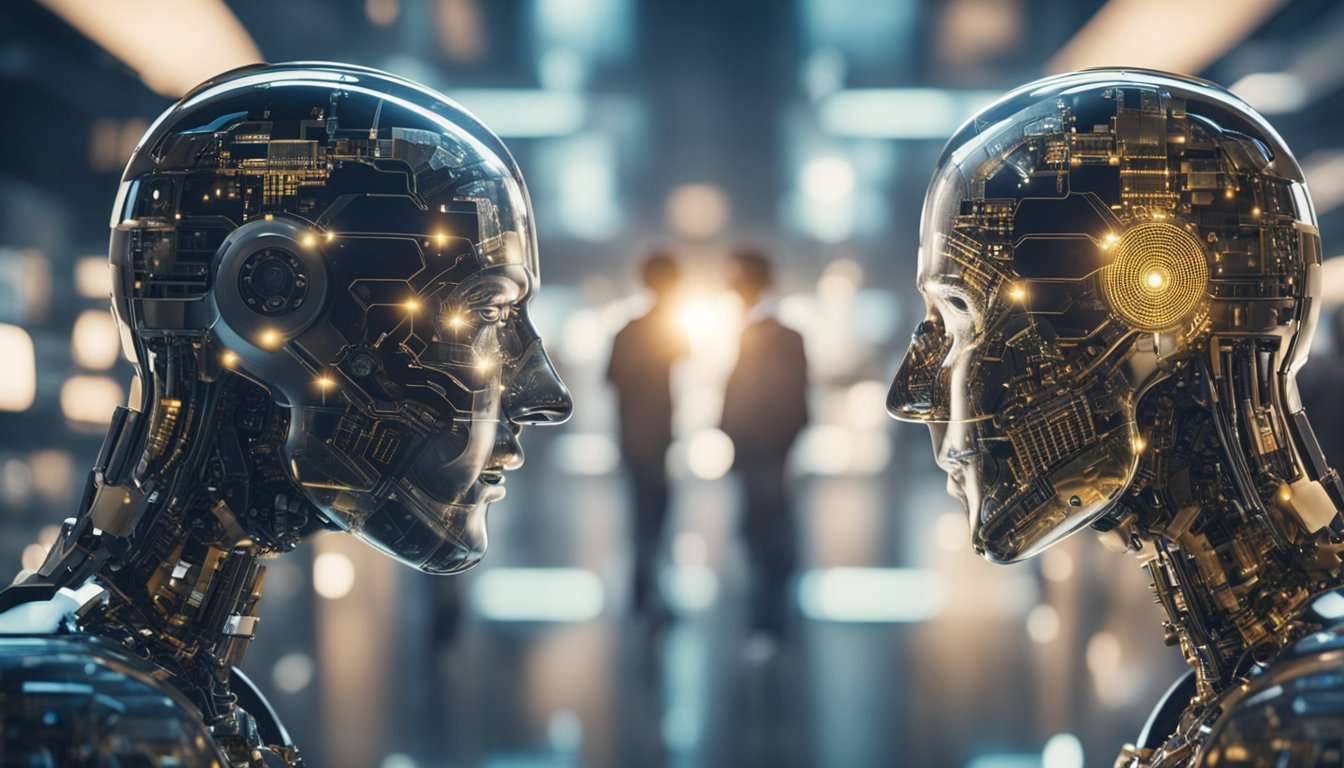
AI’s future is exciting, with both generative and predictive AI technologies playing an essential part in its shaping. Advancements have been swift in these technologies, which bodes well for their future in both areas.
One of the future trends for AI is an increase in its use for fine-tuning and improving predictive models using generative AI. Generative AI uses synthetic data synthesis to increase the training set accuracy of predictive models, leading to enhanced predictive capabilities and making them more reliable and effective than before.
Future AI trends include generative AI in graphics and other creative applications. Generative AI aims to generate new ideas, making it particularly helpful when undertaking creative tasks such as producing realistic images or videos for use across industries like gaming, entertainment and advertising.
Innovation is another driving factor behind AI’s future development. Companies are making significant investments in AI technologies and producing groundbreaking applications each day; as a result, its future looks bright, with exciting applications continually emerging for both generative and predictive AI use.
Future advancements in AI will see further integration between generative and predictive AI technologies, with each working hand-in-hand to produce more sophisticated and effective systems.
Overall, the future of both generative and predictive AI appears bright, as both technologies play a pivotal role in shaping its evolution. As AI technologies advance, we should anticipate more exciting applications of both types of artificial intelligence in the coming years.

Faisal Rafique
I’m an accomplished entrepreneur and content creator with a strong background in technology and software engineering. My expertise spans web development, eCommerce, programming, hosting management, technical support, and data science. I hold a Master’s Degree in Computer Science and Engineering, and I have over 5 years of professional experience, which I leverage to grow my digital business and popular blog, FaisalRafique.com