Generative AI vs Traditional AI: Understanding the Differences
Generative AI and Traditional AI are two categories of intelligence that have been making significant strides in the technology industry. Traditional AI, also referred to as Weak AI focuses on identifying patterns generating insights, automation and prediction. Moreover, our lives often encounter this type of AI through voice assistants, recommendation algorithms and chatbots. Generative AI is a form of artificial intelligence that generates fresh content based on learned patterns.
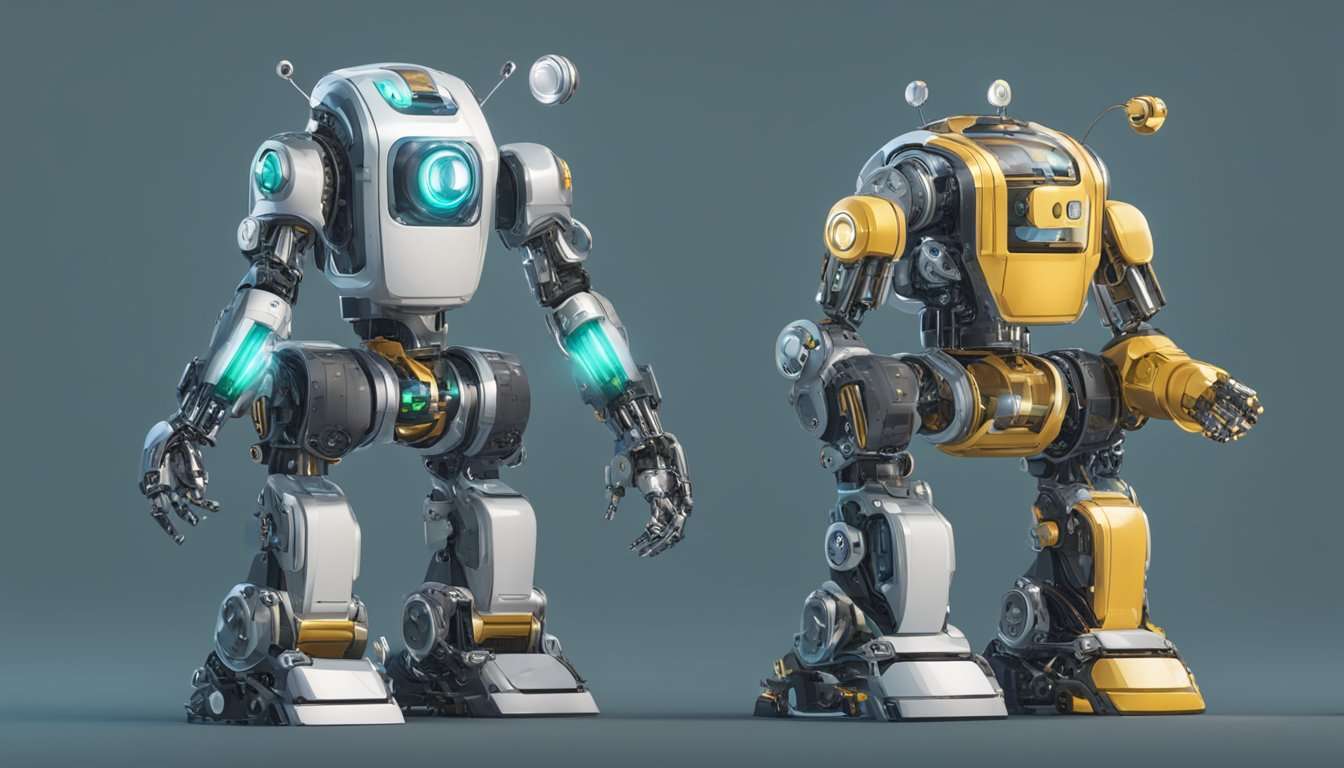
Generative AI represents a breed of AI that has gained considerable popularity in recent years. It possesses the capability to produce content, like images, videos and even text that closely resembles creations. This type of AI finds applications in industries such as music, art and literature where it aids in generating novel ideas and concepts. The technology behind Generative AI is still evolving rapidly with researchers exploring avenues, for refinement.
While both types of AI exhibit their strengths and weaknesses Generative AI holds immense promise as the future of artificial intelligence technology. By enabling the creation of content and innovative ideas it has the potential to revolutionize our perception of what can be achieved with AI and its practical applications.
As technology continues to advance we can expect to witness progress in this field and witness the implementation of Generative AI.
Key Takeaways
- Traditional AI focuses on detecting patterns generating insights, automation and prediction. On the other hand Generative AI creates content by leveraging learned patterns.
- Generative AI represents a form of artificial intelligence that has the potential to revolutionize our understanding of AI and its various applications.
- With the advancements in Generative AI technology we anticipate witnessing breakthroughs in this field and witnessing its practical use.
Understanding Traditional AI
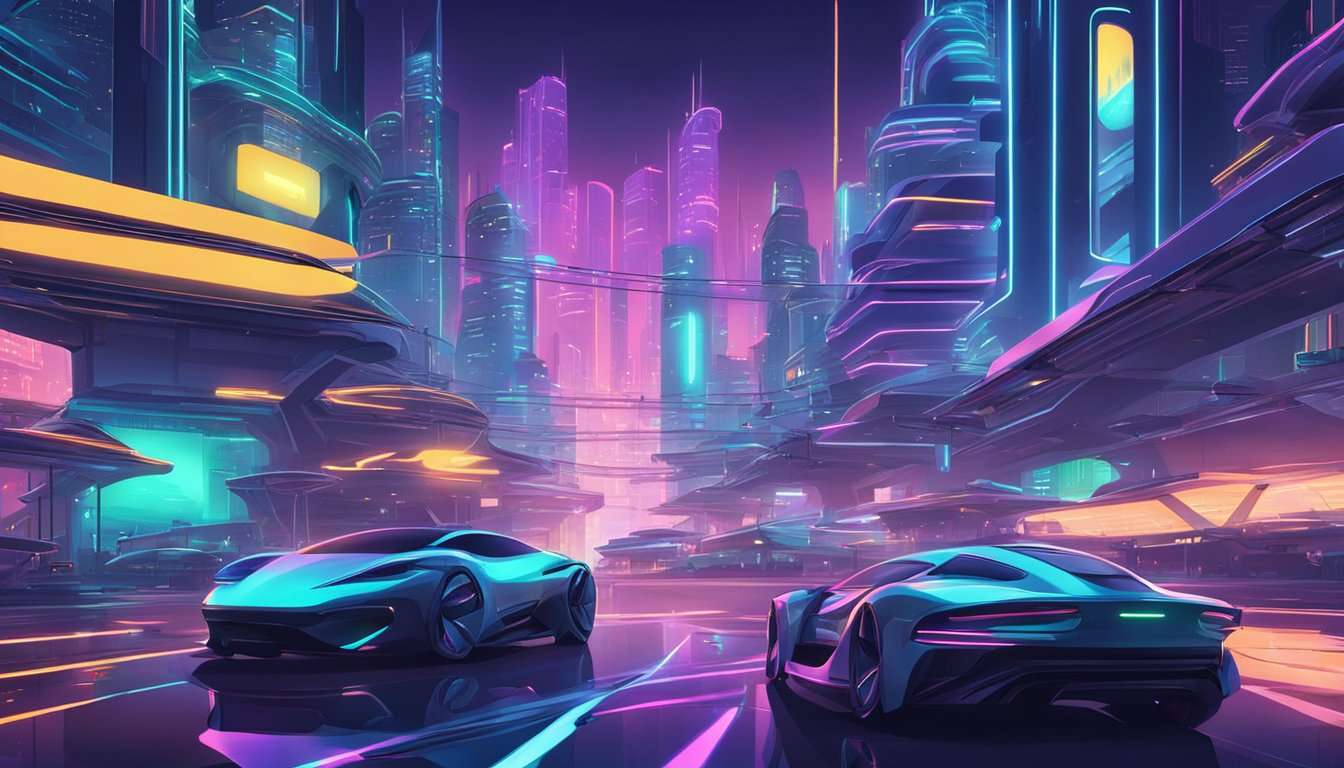
Traditional AI systems are designed to analyze data and make predictions based on that data. These systems typically operate on rule based algorithms that process data to offer insights.
Role of Algorithms in Traditional AI
Algorithms play a role in AI systems as they process vast amounts of data and identify patterns essential for making accurate predictions. These algorithms often rely on models trained with data to identify trends and patterns.
Traditional AI algorithms are usually tailored to address problems and undergo training using large datasets. This enhances their accuracy. Enables them to automate business processes while providing personalized recommendations based on users past interactions.
Applications of Traditional AI
Conventional AI finds its applications in industries. For instance in the field of finance it aids in the detection of transactions. Offers investment recommendations based on individual risk profiles. In healthcare it analyzes data to provide treatment suggestions.
Moreover e commerce extensively utilizes AI to suggest products to users by studying their purchase history. These recommendations rely on algorithms that analyze amounts of data to identify patterns and predict users future purchases.
As a whole conventional AI serves as a tool for automating business processes providing recommendations and enhancing accuracy across a wide range of applications. Nevertheless it is important to note that its predictive abilities are limited by the data it has been trained on and may not always accurately predict outcomes.
Unveiling Generative AI
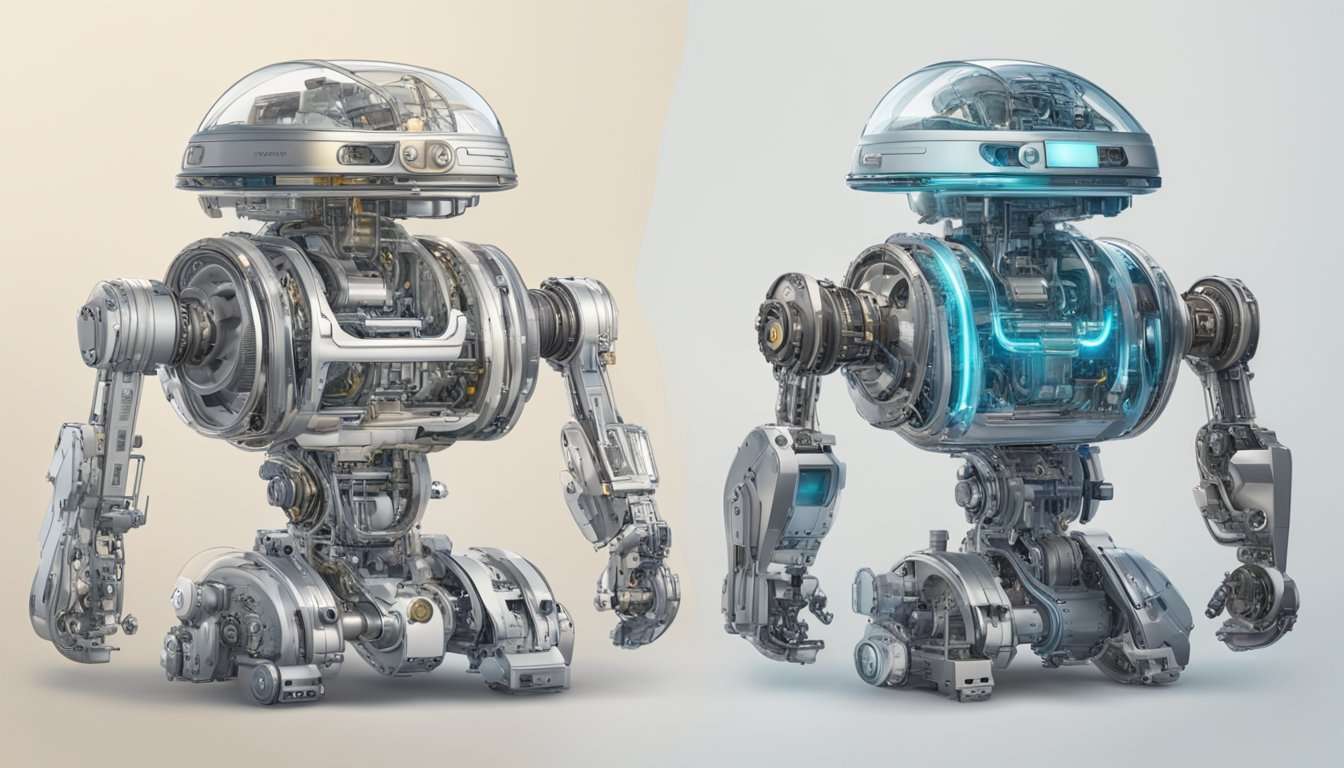
The Concept of Generative AI
Generative AI refers to an intelligence approach where machine learning models are trained to generate content resembling a given set of training data.
Unlike AI, which focuses on identifying patterns deriving insights, automation and making predictions generative AI is specifically designed to produce content, from scratch.
Generative AI finds application in industries like music, art and writing. It enables the generation of works that exhibit styles to existing ones. Furthermore it can be utilized to create products such as clothing designs or furniture pieces based on existing designs.
ChatGPT stands out as one of the known examples of generative AI. This language model generates contextually appropriate responses when provided with prompts. ChatGPT is trained using a dataset of text and employs learning techniques to generate responses that closely match the style and tone found within its training data.
Generative AI in Action: Examples
Instances where generative AI truly shines include generating images, videos and audio. For instance generative models excel at producing images depicting faces, animals and various objects by training on datasets of images; subsequently generating new visuals that resemble the style present in their training data.
Another fascinating application of AI is, in video creation. Generative models have the ability to be trained on collections of video footage. They can then generate videos that share a style to the training data. This technology finds its applications, in the film industry, where it aids in crafting effects and other visual elements.
Moreover generative AI can also be utilized for creating music and other audio content. By training models on collections of music they can generate songs that reflect the style of the training data. This technology has implications in the music industry enabling the creation of compositions and remixes.
In essence generative AI is a tool that is revolutionizing content creation and consumption. As advancements continue to be made in this field we can anticipate innovative applications of generative AI in the years ahead.
Distinguishing Features of Generative AI and Traditional AI
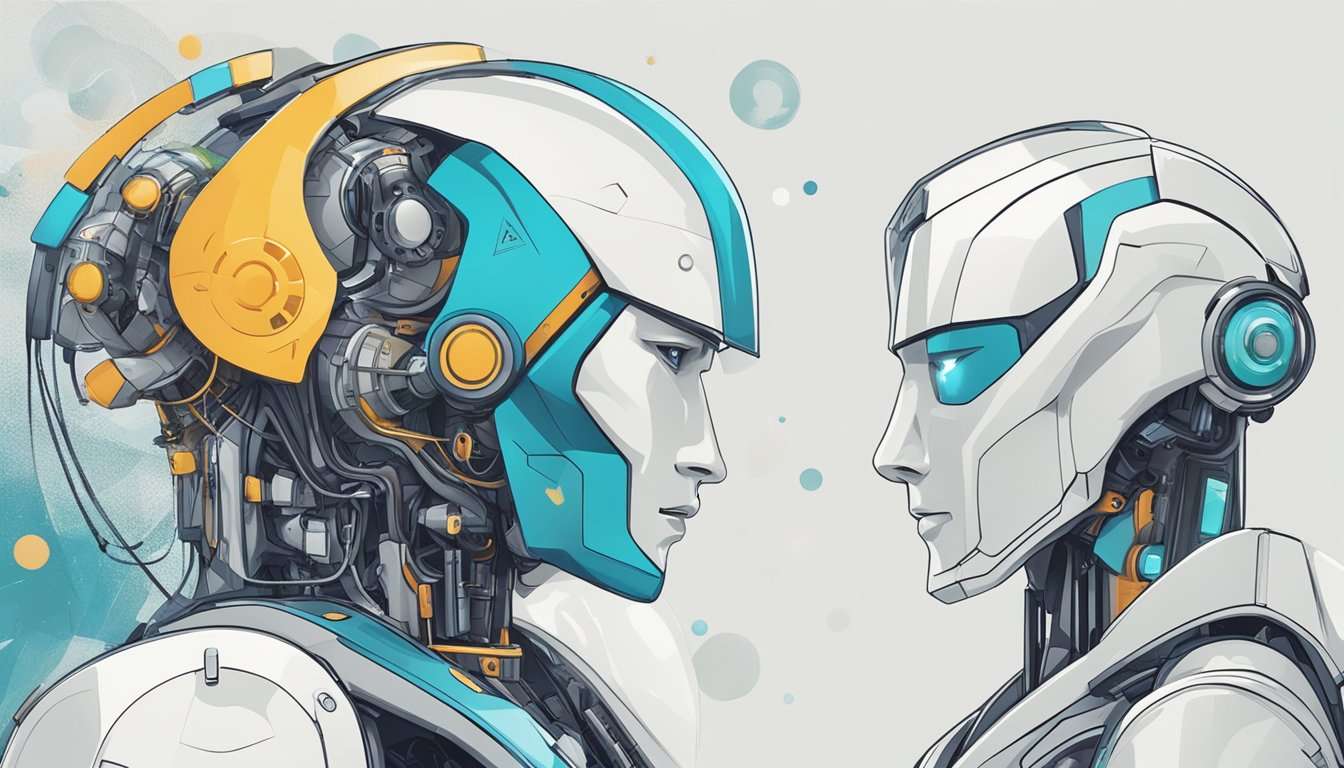
Comparing Performance
When comparing Generative AI with Traditional AI it becomes evident that they possess characteristics. Traditional AI is designed to execute tasks using defined algorithms and rules. It is often referred to as Narrow AI or Weak AI. Conversely Generative AI has the capability to generate content based on patterns, within data without requiring intervention.
Generative AI has demonstrated performance and innovation compared to Traditional AI. Its versatility and adaptability make it highly useful, in fields as it can autonomously generate text, images and music. Furthermore Generative AI has the ability to learn from data and generate ideas, a capability that Traditional AI lacks.
Examining Limitations
However it is important to acknowledge the limitations of Generative AI. It heavily relies on an amount of data to produce results which can be challenging in certain industries. Additionally there is a concern regarding security as Generative AI has the potential to generate content that closely resembles materials.
On the hand Traditional AI has its set of limitations with regards to innovation and adaptability. It is primarily designed for tasks without the capacity, for learning from data or generating concepts. Moreover the accuracy of Traditional AI can be influenced by the quality of training data it receives.
In conclusion while both Generative AI and Traditional AI possess strengths and weaknesses that suit their purposes their distinctions enable them to excel in various domains.
Generative AI offers versatility and adaptability making it highly valuable, in industries. On the hand Traditional AI is better suited for tasks that require high levels of accuracy.
The Role of Large Language Models in Generative AI
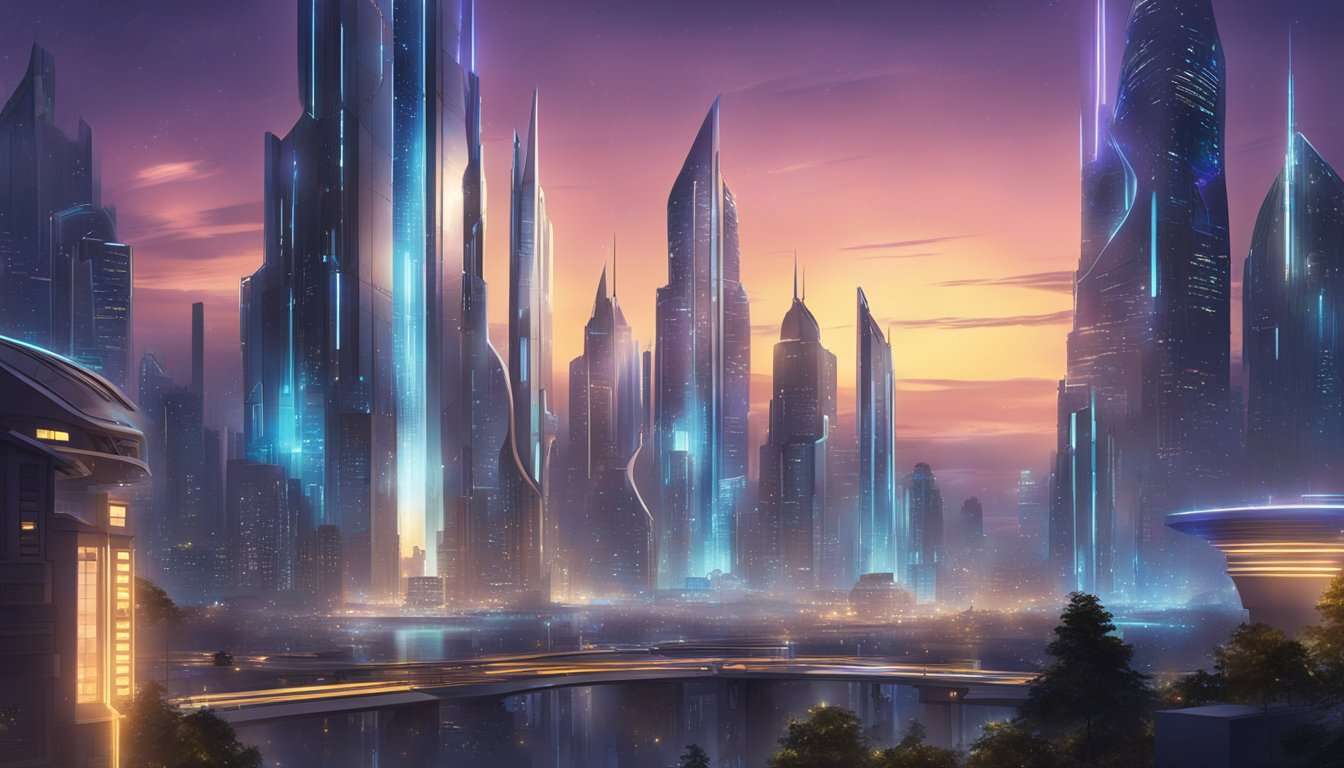
Generative AI encompasses a range of intelligence techniques that involve generating new content using Large Language Models (LLMs) and other types of AI models. LLMs are specifically designed to work with language and aim to create simplified yet digital representations. In this section we will explore the significance of LLMs in Generative AI by discussing their functionality and various applications.
Understanding Large Language Models
LLMs are developed based on text data sets used for training purposes. These data sets can consist of books, articles, social media posts or any other collection of information. The size of the data set directly influences the accuracy level of the LLM. By training on billions of parameters LLMs can predict the probability distribution for word sequences based on preceding words.
Applications of Large Language Models
Language Models find applications in diverse areas such, as chatbots, language translation and content generation. Prominent examples include OpenAIs ChatGPT and Google Bard both leveraging LLM technology.
These chatbots have the ability to comprehend language and generate responses that closely resemble those of a human. Language generation models (LLMs) are also utilized in language translation applications enabling them to translate text from one language to another.
In the realm of content generation LLMs can be employed to generate text based on a given prompt. For instance an LLM can be trained on a collection of news articles. Then utilized to produce articles centered around a specific topic. LLMs also aid in uncovering insights, into language including identifying patterns and trends in language usage.
All LLMs play a role in the field of Generative AI by serving as a foundation for natural language processing. By comprehending the significance of LLMs in Generative AI we can develop an understanding of its capabilities and limitations.
Future of AI: Generative vs Traditional
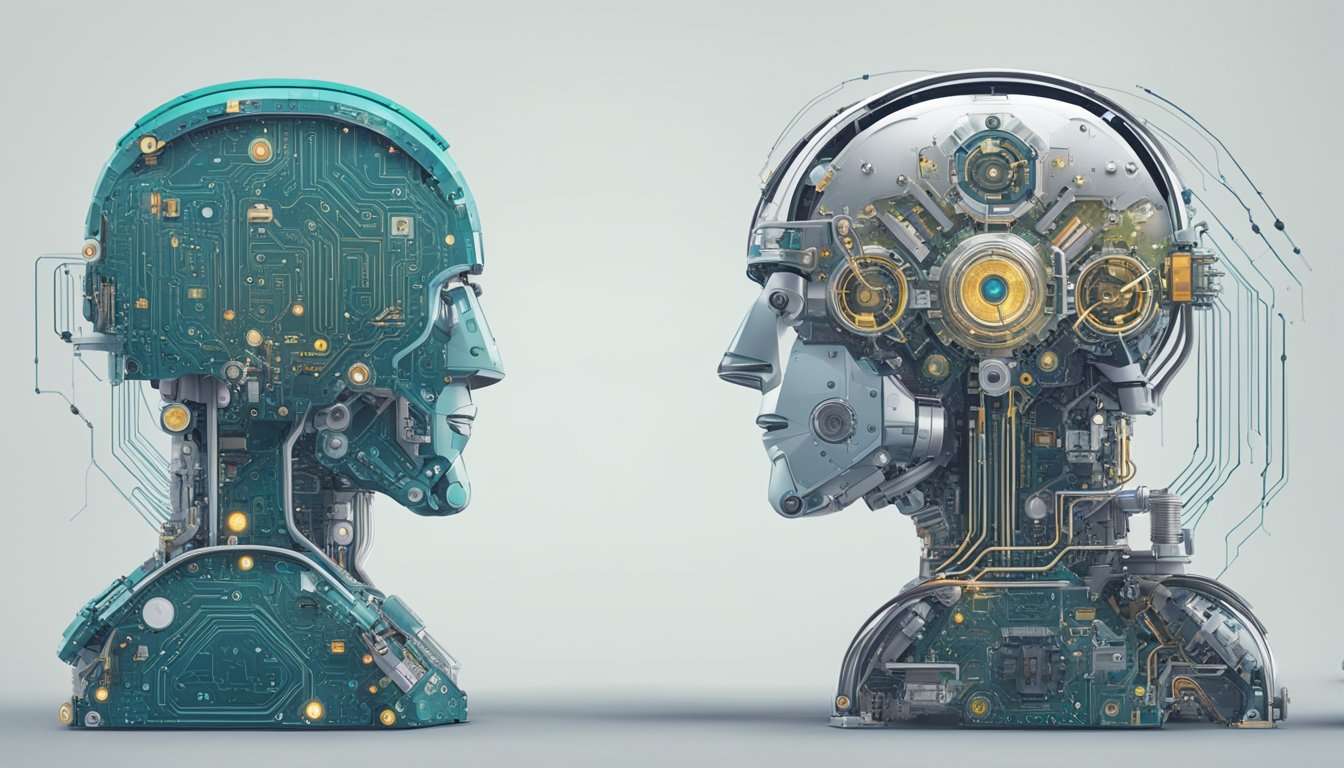
The prospects for AI are incredibly promising offering boundless opportunities for innovation. There are two approaches currently being pursued; AI and traditional AI. Traditional AI, also referred to as weak AI focuses on intelligently performing specific tasks such as voice assistants, like Siri or Alexa recommendation engines employed by Netflix Amazon or Google’s search algorithm.
On the side generative AI represents a sophisticated form of artificial intelligence that can generate fresh content, like text, images or even music by learning from existing data. It all begins with a prompt where users can submit a question along with any information to guide the content creation process. The ability of AI lies in its ability to revolutionize industries which include art, enjoyment and advertising with the aid of producing customized content that caters to the particular wishes of customers.
One major advantage of AI compared to AI is its capacity to learn and adapt to new situations. Traditional AI is constrained by the data it has been trained on and struggles with handling novel scenarios it hasn’t encountered before. On the hand generative AI can absorb data and produce unique content that aligns precisely with user requirements.
Although traditional AI currently dominates applications due to its approach and reliability generative AI is rapidly progressing and holds great potential for transforming our interaction with technology. The inclusion of AI is projected to boost compound growth rates (CAGR) by an average of 0.5 to 0.7 percentage points for early adopters and 0.1 to 0.3 percentage points, for late adopters.
In conclusion the future of AI appears promising as both generative AI and traditional AI play roles, in applications. While traditional AI is widely used and reliable in settings generative AI has the potential to revolutionize industries like art, entertainment and marketing by creating personalized content that meets users specific needs.

Faisal Rafique
I’m an accomplished entrepreneur and content creator with a strong background in technology and software engineering. My expertise spans web development, eCommerce, programming, hosting management, technical support, and data science. I hold a Master’s Degree in Computer Science and Engineering, and I have over 5 years of professional experience, which I leverage to grow my digital business and popular blog, FaisalRafique.com